The work of data science teams can be intertwined with cloud and other tech assets, which can make them part of budgetary questions raised about cloud spending. This is just one of the ways data scientists have expanded beyond some old expectations of the work they do and the assets they leverage. If steps are not taken to sort out how such resources are used, organizations might see data science contribute more to costs rather than returns.
Shane Quinlan, director of product management with Kion, spoke with InformationWeek about how data science has evolved, and ways data scientists can efficiently use the cloud.
Are data scientists working outside the box compared with what has been expected of them? What different angles are they taking to fulfill their duties?
Data science wasn’t something really on my radar when I started working in technology. The buzz started in 2015-2018, when data science become the thing. New positions started getting created and we started getting things like DataOps and MLOps. Big data--if you slap that onto any company, then gold mine.
I got pulled into it around that same timeframe, moving from a job where I was working, mostly supporting federal and law enforcement customers, jumping into healthcare. Switching from web and endpoint solutions to analytics. That was my first jump into data science.
Now I’m seeing it from a different angle because our product focus is much more on platform and infrastructure management. I’m looking at it from the cloud towards data science instead of looking at from data science towards the cloud.
What are the influences and factors that affect the approaches that data scientists take? As data scientists leverage the cloud, what do they need to be more mindful of?
I see two trends. One is around changes in technology and availability. Early on, it was kind of the Wild West. There were tons of new service offerings, technology stacks, and the skillsets were really divergent and started to be a little bit more accessible.
Data science was this big world. You had everything from your Excel data scientist literally using Microsoft Excel, to an expectation that you could write Java applications that could perform data functions and provide different output. You had mathematicians, you had statisticians, you had software developers, and you had folks who had more of a business intelligence-analyst role all coming at the same space and trying to find different ways to meet their expectations.
That’s when you saw a push for better user interfaces, making the development side less of a requirement. That’s where you have the introduction of notebooks like Jupyter and Zeppelin and derivations thereof to make that a little bit easier. You had like a human interpretable code and not-code interface with the way that you’re shaping data. Behind the scenes, I think there’s been this huge explosion of ways to shape that as well. You have tech like DBT that’s making the data transformations a lot easier. Technologies that were centered around the Apache Hadoop ecosystem have now shifted and morphed and moved all over the place making it a lot more portable. Apache Spark can be run in all kinds of different contexts now.
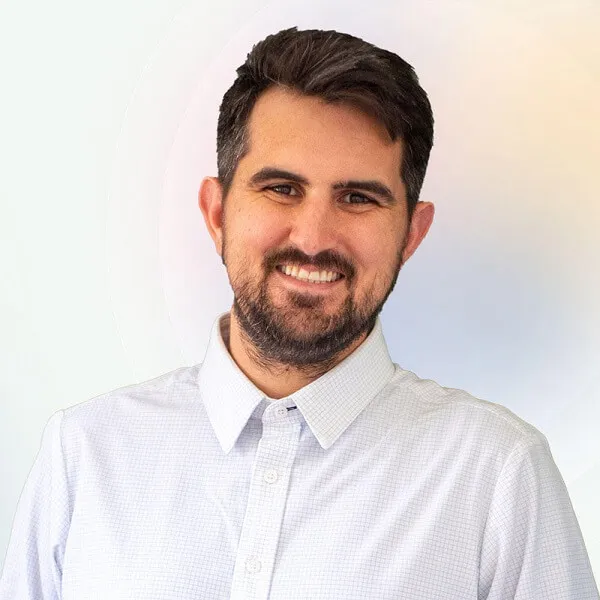
There’s been a drive towards a more user-centric model of data science. More user-friendly, more user interfaces, more easily interpretable. You can bring common skillsets like Excel or BI tools or SQL and do enough with that to make a difference.
The other side of that is a development-centric approach, where as a developer it makes data science more approachable versus asking mathematicians to learn to be developers.
Another piece is this tension around bigness and just how much data is required to create the kinds of insights you need to provide business value. The CEO of Landing AI [Andrew Ng] has made this huge push for 'big datasets are dumb'. [Big datasets are] wasting money, they’re wasting time. Cleaner, smaller datasets are actually more impactful. [Ng has said you don’t always need “big data,” but rather “good data.”] You see this tension between the traditional approach of 'get all the data and learn as much as you can from it,' versus cleaner, smaller less expensive, more efficient datasets providing that insight.
Some of it comes back to folks trying to do magic with what they had. Way too many I’ve talked to were like, “We have all this data; we need to do something with it.”
Okay. Great. What?
And they’d say, “Well, we need to run some machine learning so we can see what we can find out.”
It doesn’t work that way. You have to bring an actual scientific mindset to understand what hypothesis you are testing by using these models. It requires a very specific mindset to have that much discipline and the way you approach problem-solving and value creation through data science techniques versus, 'I have data; please do things.'
When IT budgets come under scrutiny with data scientists making use of the cloud, what can be done to sort out their organization’s needs?
The great thing about cloud is you use it when you need it. Obviously, you pay for using it when you need it but often times data science applications, especially ones you’re running over large datasets, aren’t running continuously or don’t need to be structured in a way that they run continuously. Therefore, you’re talking about a very concentrated amount of spend for a very short amount of time. Buying hardware to do that means your hardware sits idle unless you are very active about making sure you’re being very efficient in the utilization of that resource over time.
One of the biggest advantages of cloud is that it runs and scales as you need it to. So even a tiny can run a massive computation and run it when they need to and not consistently.
That adds challenges, of course. “I fired this thing off on Friday, I come back in on Monday and it’s still running, and I accidentally spent $6,000 this weekend. Oops.” That happens all the time and so much of that is figuring out how to establish guardrails.
Sometimes data science gets treated like, “You know, they’re going to do whatever they need to.”
In the development world, we’ve started to have language to speak to this risk-taking, experimental, 'don’t punish failure, we learn from failure'. We’ve been able to bring that language in, but we’ve ignored data science.
Are there some best practices for balancing and managing the innovations data scientists might want to take advantage of?
If your data science department is young and small, cloud-first sounds scary but will set you up for success down the line. If you want to make those choices on hardware investments, then you can make them at the appropriate time instead of thinking you need to buy hardware upfront and then go to cloud later, which is infinitely harder.
Guardrails don’t have to be rocket science. They can be simple. Simple can be very effective.
What to Read Next:
An Insider's Look at Intuit's AI and Data Science Operation